Penn Researchers Solve a Decades-old Question About Glass Transitions
If one were to take a liquid — any liquid — and cool it down rapidly enough so that it doesn’t have a chance to crystallize, the result would be glass. Glass is so viscous that it takes too long to flow for anyone to realize that it is liquid rather than solid.
“We would never notice glass flow in our lifetimes or in a billion of our lifetimes or even hundreds of billions,” said Andrea Liu, Hepburn Professor of Physics in the School of Arts & Sciences at the University of Pennsylvania. “Even in many, many times the lifetime of the universe. There are just ridiculous timescales involved here.”
Liu added that this is true of every liquid, and they all do it in very similar ways.
But how this process works has puzzled scientists ever since they realized it was happening. One huge question that remains is whether structure is connected to dynamics when it comes to these glass transitions.
“If you look at the viscosity, you can see it changing by ten orders of magnitude when you cool by only tens of degrees,” Liu said. “That’s called looking at the dynamics. But, if you look at the actual structure, at how the molecules are arranged, you'll hardly see any change at all. That’s one of the reasons why people had largely given up on finding any connection between structure and dynamics.”
In a paper published in the Proceedings of the National Academy of Sciences, researchers at Penn and Harvard University solved this decades-old question using an unconventional method: machine learning.
The study was a result of collaboration between Penn Ph.D. graduate Samuel Schoenholz, who is now a Brain Resident at Google, and Harvard Ph.D. graduate Ekin Dogus Cubuk, who is now a postdoc at Stanford University. Penn’s Liu and Efthimios Kaxiras, a professor of physics at Harvard, were the students’ faculty advisors for the research.
To show that dynamics and structure are related, Schoenholz and Cubuk came up with the idea of applying machine learning.
According to Liu, breakthroughs in machine learning are what suddenly enabled advances like Google Translate and “intelligent assistants” such as Siri.
“It's basically a way to take a lot of different information and synthesize it to be able to do things like recognize faces or navigate a car,” said Liu.
Although many machine learning algorithms, such as the one that enabled Google Translate, are extremely complex, the one used in this research was very straightforward and easy to understand.
The traditional physics approach to understanding this question has been to identify a structural quantity that might be important and then to see if it controls the dynamics.
The machine learning technique, on the other hand, takes hundreds of quantities that individually might not be expected to have much correlation, and then it finds the combination of these quantities that correlates strongly with the dynamics.
Although machine learning hasn’t really been a technique that has been used for this purpose in the physical sciences, Liu said, the researchers wouldn’t have been able to reach these results without it.
“This is one of the few examples where we've gained really new conceptual understanding into a problem that we really couldn't make progress on in another way, and the machine learning was the key to let us do this,” Liu said.
This new technique is vital when it comes to understanding systems that are far from equilibrium.
When it comes to systems in thermal equilibrium, for example, physicists have developed an extremely powerful formalism called statistical mechanics that allows them to study the collective properties of many, many particles, or many-body systems.
The behavior of an equilibrium liquid depends on just a few variables: temperature, pressure and density. That’s really all one needs to specify: if they know these quantities, then the properties of the liquid are ordained.
But this fails in out-of-equilibrium systems such as glass. Until now, scientists thought they would need to specify a lot more detailed information when it came to glass than they would need to specify for a system that’s in equilibrium, such as the history of how the glass was prepared.
The researchers showed that, in fact, they don't need to specify all of that. All they need are the usual things, plus one: the machine learning derived quantity, which they called softness. If softness is known, Liu said, the behavior of the glass can be predicted.
Using machine learning, the researchers were able to find the structural signature of the dynamics and follow it. Their results show that they can make a very simple connection between the relaxation time, which is directly related to the viscosity, and the structure, as quantified by softness, through a very simple equation that seems to describe what's going on.
One possible application for this research that Liu and several others are working on as part of Penn’s Laboratory for Research on the Structure of Matter is using similar ideas to understand the process that leads disordered solids to mechanical failure.
“We want to apply this to systems that are under mechanical load to learn how to predict failure, or how the system’s going to fail,” Liu said.
Another direction the researchers hope to take this research is to get a better understanding of the glass transition.
Liu said that the biggest challenge in the project was just to think of applying machine learning in the first place.
“Once Sam and Dogus had the machine learning and figured out how to do that, I'd say the results sort of fell out,” she said.
The research was supported by a grant from the Department of Energy and Simons Foundation.
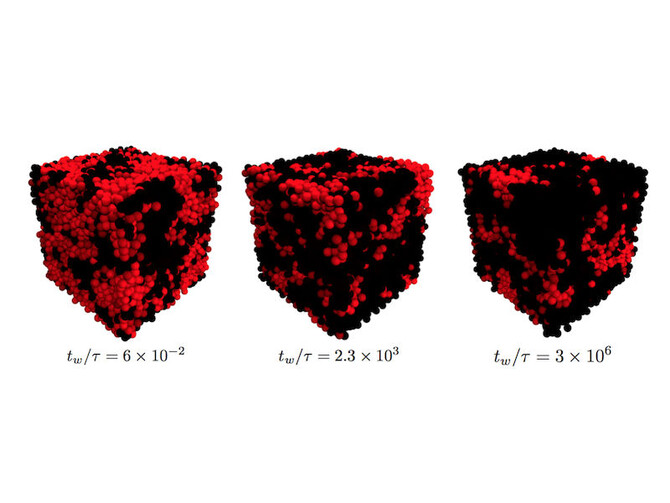