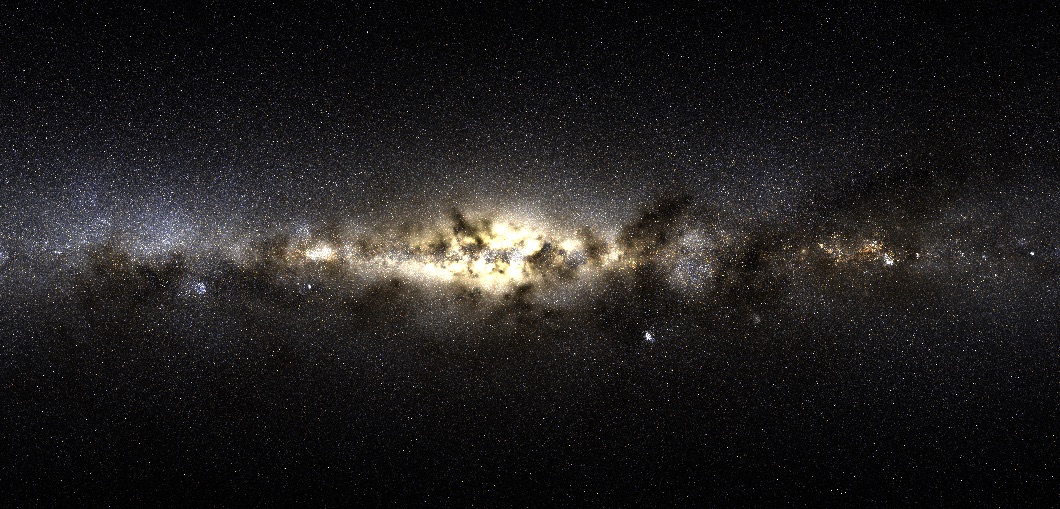
Researchers have discovered a new cluster of stars in the Milky Way disk, the first evidence of this type of merger with another dwarf galaxy. Named after Nyx, the Greek goddess of night, the discovery of this new stellar stream was made possible by machine learning algorithms and simulations of data from the Gaia space observatory. The finding, published in Nature Astronomy, is the result of a collaboration between researchers at Penn, the California Institute of Technology, Princeton University, Tel Aviv University, and the University of Oregon.
The Gaia satellite is collecting data to create high-resolution 3D maps of more than one billion stars. From its position at the L2 Lagrange point, Gaia can observe the entire sky, and these extremely precise measurements of star positions have allowed researchers to learn more about the structures of galaxies, such as the Milky Way, and how they have evolved over time.
In the five years that Gaia has been collecting data, astronomer and study co-author Robyn Sanderson of Penn says that the data collected so far has shown that galaxies are much more dynamic and complex than previously thought. With her interest in galaxy dynamics, Sanderson is developing new ways to model the Milky Way’s dark matter distribution by studying the orbits of stars. For her, the massive amount of data generated by Gaia is both a unique opportunity to learn more about the Milky Way as well as a scientific challenge that requires new techniques, which is where machine learning comes in.
“One of the ways in which people have modeled galaxies has been with hand-built models,” says Sanderson, referring to the traditional mathematical models used in the field. “But that leaves out the cosmological context in which our galaxy is forming: the fact that it’s built from mergers between smaller galaxies, or that the gas that ends up forming stars comes from outside the galaxy.” Now, using machine learning tools, researchers like Sanderson can instead recreate the initial conditions of a galaxy on a computer to see how structures emerge from fundamental physical laws without having to specify the parameters of a mathematical model.
The first step in being able to use machine learning to ask questions about galaxy evolution is to create mock Gaia surveys from simulations. These simulations include details on everything that scientists know about how galaxies form, including the presence of dark matter, gas, and stars. They are also among the largest computer models of galaxies ever attempted. The researchers used three different simulations of galaxies to create nine mock surveys—three from each simulation—with each mock survey containing 2-6 billion stars generated using 5 million particles. The simulations took months to complete, requiring 10 million CPU hours to run on some of the world’s fastest supercomputers.
The researchers then trained a machine learning algorithm on these simulated datasets to learn how to recognize stars that came from other galaxies based on differences in their dynamical signatures. To confirm that their approach was working, they verified that the algorithm was able to spot other groups of stars that had already been confirmed as coming from outside the Milky Way, including the Gaia Sausage and the Helmi stream, two dwarf galaxies that merged with the Milky Way several billion years ago.
In addition to spotting these known structures, the algorithm also identified a cluster of 250 stars rotating with the Milky Way’s disk towards the galaxy’s center. The stellar stream, named Nyx by the paper’s lead author Lina Necib, would have been difficult to spot using traditional hand-crafted models, especially since only 1% of the stars in the Gaia catalog are thought to originate from other galaxies. “This particular structure is very interesting because it would have been very difficult to see without machine learning," says Necib.
But machine learning approaches also require careful interpretation in order to confirm that any new discoveries aren’t simply bugs in the code. This is why the simulated datasets are so crucial, since algorithms can’t be trained on the same datasets that they are evaluating. The researchers are also planning to confirm Nyx’s origins by collecting new data on its stream’s chemical composition to see if this cluster of stars differs from ones that originated in the Milky Way.
For Sanderson and her team members who are studying the distribution of dark matter, machine learning also provides new ways to test theories about the nature of the dark matter particle and where it’s distributed. It’s a tool that will become especially important with the upcoming third Gaia data release, which will provide even more detailed information that will allow her group to more accurately model the distribution of dark matter in the Milky Way. And, as a member of the Sloan Digital Sky Survey consortium, Sanderson is also using the Gaia simulations to help plan future star surveys that will create 3D maps of the entire universe.
“The reason that people in my subfield are turning to these techniques now is because we didn’t have enough data before to do anything like this. Now, we’re overwhelmed with data, and we’re trying to make sense of something that’s far more complex than our old models can handle,” says Sanderson. “My hope is to be able to refine our understanding of the mass of the Milky Way, the way that dark matter is laid out, and compare that to our predictions for different models of dark matter.”
Despite the challenges of analyzing these massive datasets, Sanderson is excited to continue using machine learning to make new discoveries and gain new insights about galaxy evolution. “It’s a great time to be working in this field. It’s fantastic; I love it,” she says.
Robyn Sanderson is an assistant professor in the Department of Physics and Astronomy in the School of Arts & Sciences at the University of Pennsylvania.
Gaia is a space observatory of the European Space Agency whose mission is to make the largest, most precise three-dimensional map of the Milky Way Galaxy by measuring the positions, distances, and motions of stars with unprecedented precision.
Supercomputers used for this research included Blue Waters at the National Center for Supercomputing Applications, NASA's High-End Computing facilities, and Stampede2 at the Texas Advanced Computing Center.