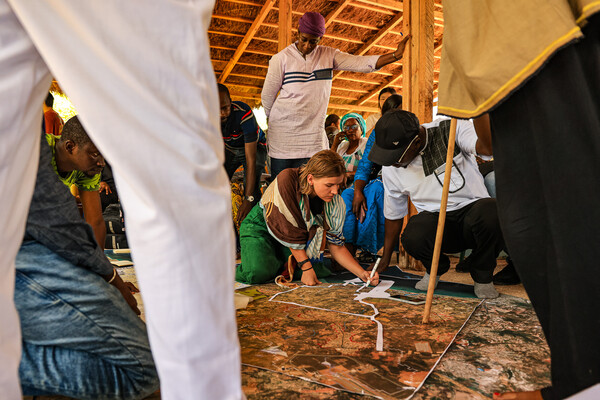
In Senegal, the ambitious Dakar Greenbelt project seeks to create an extensive network of ecological infrastructure in and around the city to sustainably address environmental concerns and enhance urban life. With support from David Gouverneur and Ellen Neises, Ph.D. candidate Rob Levinthal in the Weitzman School of Design led two courses that included a field trip to Dakar, that culminated in students presenting their visions for parts of the Greenbelt.
(Image: Courtesy of Chaowu Li)