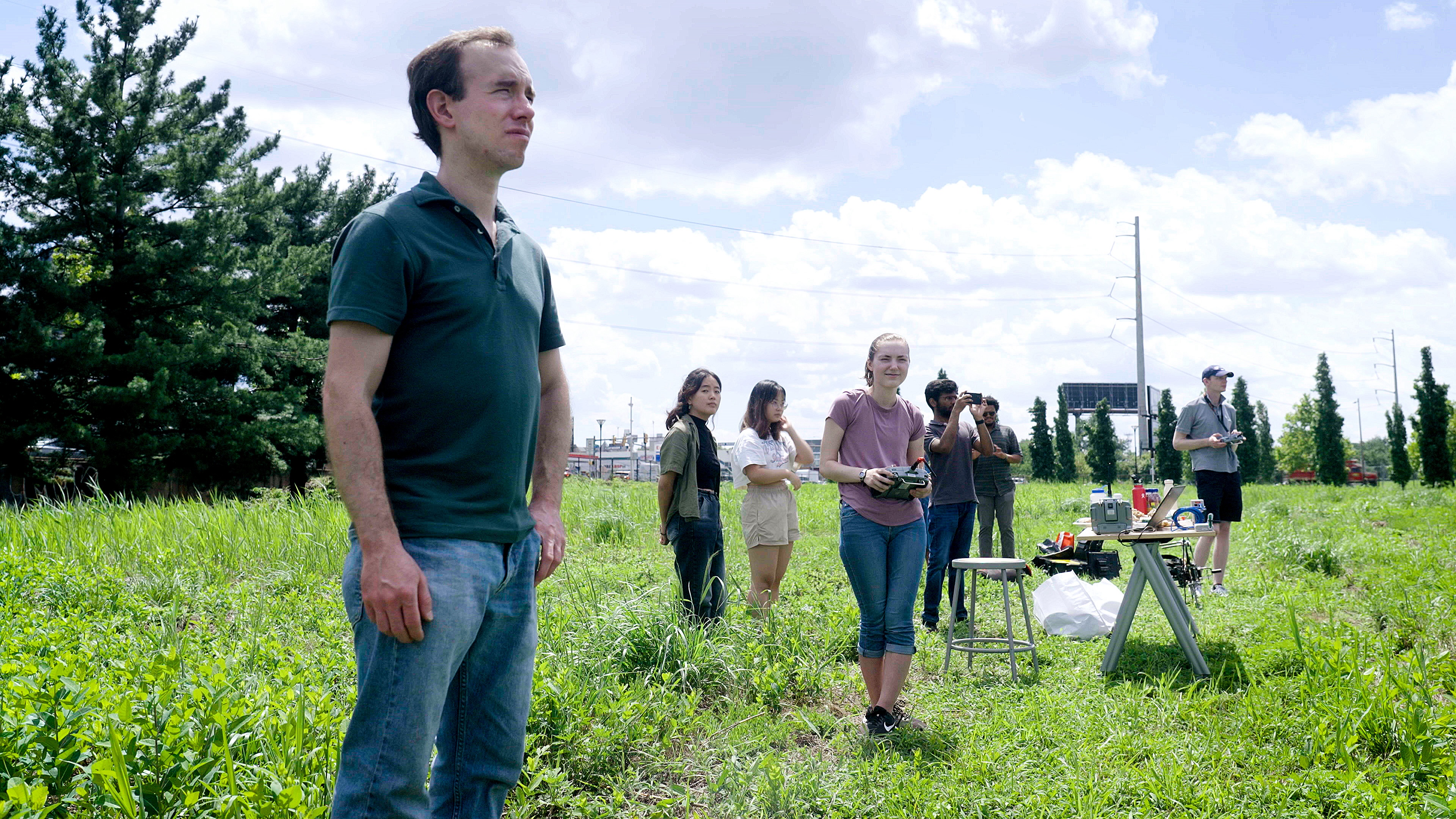
On Feb. 6, 2023, massive earthquakes struck Turkey and Syria. Measuring 7.8 and 7.7 on the Richter scale and felt as far away as Egypt, the temblors damaged an area the size of Germany. The disaster affected nearly one-sixth of Turkey’s population, the equivalent of a single natural disaster impacting 48 million Americans.
In the immediate aftermath, first responders faced a dilemma. With so many collapsed buildings, where should they focus their efforts? Local emergency services were overwhelmed. The critical window for finding victims after an earthquake is 72 hours, any longer and survivors may perish from a lack of food, water, and medical care.
“Triage, I think the root of the word is sorting,” says Jeremy Cannon, professor of surgery at the Perelman School of Medicine and an Air Force veteran who deployed to Iraq and Afghanistan as a trauma surgeon. “You’re trying to sort patients to identify who will benefit from immediate therapy, who is going to make it and survive regardless of what you do.”
And, of course, there is the fact that part of triage is deciding who is too far gone to save. “This is traditionally challenging when there is gunfire or explosions, or in the setting of, say, an earthquake,” Cannon says. “It’s often fraught with danger, so first responders are in many instances putting themselves at risk to perform this initial sorting of patients.”
Last year, the Defense Advanced Research Projects Agency (DARPA), the research arm of the Pentagon, announced a challenge to spur innovation in triage, specifically for mass casualty incidents (MCI), which by definition overwhelm local health care systems. “DARPA wants teams to develop ways to use robots and drones to help with the triage process,” Cannon says. “It’s sort of futuristic, almost science fiction, because it involves not just identifying injuries but assessing vital signs and patient physiology.”
Penn Robotic Non-contact Triage and Observation (PRONTO) team is brings together experts in trauma from Penn Medicine and experts in robotics and computer vision from the School of Engineering and Applied Science and the General Robotics, Automation, Sensing, and Perception (GRASP) Laboratory.
“I’m super impressed that we even have a spot at the table,” Cannon says. “But I think that really speaks to Eric’s reputation for excellence and his innovative mind and the great team that he’s been able to assemble.”
“The goal,” Eric Eaton says, “is when something like this happens the first responders roll up, press a big red button, and off go a couple of ground robots and aerial robots to survey the area.” Eaton is a research associate professor in computer and information science (CIS) at Penn Engineering, a core faculty member of GRASP Lab, and PRONTO’s principal investigator.
Planning for worst-case scenarios
In 2021, Penn Medicine joined the U.S. Navy in a unique partnership, bringing active duty trauma surgeons to Penn Presbyterian Medical Center. “The basic idea is that, between deployments, they want to make sure that the trauma team has skills that are kept up to date,” Eaton says. The partnership has created an environment where Penn trauma surgeons can learn from active-duty service members and vice versa.
The partnership—the first of its kind—means that Penn Medicine can help develop the next generation of triage techniques. Cannon points to Emergency Medicine’s Zaffer Qasim as “a vital point person for guiding and informing the team on how first responders and pre-hospital personnel approach triage in real-world disaster scenarios.”
Qasim serves as a source of feedback for the roboticists involved in the project. “His insights in emergency medicine and trauma help make sure that the team’s technological solutions are still grounded in the practical realities that first responders face on the ground,” Cannon says.
Jay A. Yelon, one of the Navy trauma surgeons placed at Penn, has also played an integral role in PRONTO. “Together, Zaffer, Jay, and I are pooling our diverse medical experiences to create realistic practice scenarios for the engineers, allowing them to simulate and test the robotics platform,” Cannon says.
Integrating trauma best practices into PRONTO involves being ready for predictable failure but also considering less-than likely scenarios in planning responses to MCIs. “Traditionally, the weak link in disaster preparation is our inability to imagine what could happen and what it would look like when we’ve never seen it before,” Cannon says.
As a result, successful triage planning often favors imagining the unimaginable. “We decided to do an exercise and invest a lot of resources, time, and effort to prepare our team,” Cannon says of an instance where the Pentagon prepared for an MCI following an airplane crash, prior to 9/11. A decade later, victims of the Boston Marathon bombing benefited because Brigham and Women’s Hospital planned for such an unlikely event. What would it be like if they had many trauma patients come in all at once, some in need of tourniquets?
In both cases, first responders eventually saved lives because plans had been made for these exact scenarios, emphasizing the importance of preparation in successful triage. Cannon says. “It’s uncomfortable to consider such devastating events, but that discomfort doesn’t make the risk any less real. It behooves us to be ready.”
Machine learning meets medicine
For the past year, Eaton has been working to turn PRONTO’s dream of a robotic triage system into reality, working with his GRASP Lab colleagues Kostas Daniilidis, Ruth Yalom Stone Professor in CIS; Camillo J. Taylor, Raymond S Markowitz President’s Distinguished Professor in CIS; and a mix of undergraduate, master’s, and doctoral students, including Jason Hughes and Marcel Hussing, who focused on robotic systems and machine learning, respectively.
At present, PRONTO involves two wheeled ground robots, which look like tiny cars, and one drone. The team of robots is designed to fan out across a disaster area and collect data. “My background is in AI and machine learning,” Eaton says. “I work specifically on learning systems that are deployed for extended periods of time in an environment and also systems that interact or collaborate to get a solution.”
To that end, the robots in PRONTO have multiple means of studying their environment. One is “human-aware pose modeling,” a set of techniques that involves estimating the position of the human body based on visual data. “This is relatively easy if you’re in a big open grassy field and the person is wearing a contrasting color of clothing,” Eaton says. “It is much harder if they’re half-buried in rubble.”
One way to solve this problem is to train the robots using AI, teaching them to synthesize information like if a body is moving or static, emitting sound, or silent, bleeding or not. “We’re doing this with deep compositional networks,” Eaton says, referring to a species of AI that focuses on the relationship between an object and its constituent parts. “We’re building them to combine data with medical knowledge to measure things like the respiration rate, without touching the patient, and to diagnose whether or not there’s evidence of injuries like severe hemorrhage and respiratory distress.”
In addition to standard cameras, LIDAR, thermal imaging cameras, and microphones, some of the robots are also equipped with a relatively new type of sensor, known as a neuromorphic camera or event camera, that detects changes in light intensity. Compared to normal cameras, which capture, say, 120 frames per second, event cameras collect millions of pieces of data per second, since each pixel is treated independently, allowing the camera to visualize motion at tiny scales, like the vibration of a tuning fork. Even in near darkness, these cameras can pick up details like the flow of blood, Eaton says. “You can also detect things like respiration rate because it involves movement.”
In essence, the robots collectively serve as the extended eyes and ears of first responders. “All of these data points are combined together to get a clinical signature,” Eaton says. “That signature can then be used in terms of standard protocols that allow you to figure out, based on certain injuries, what level they should be triaged as and also to recommend lifesaving interventions.”
PRONTO plots the position of those clinical signatures in physical space. In tests, the robots generated maps of the Penn Engineering Research and Collaboration Hub branch of the GRASP Lab, at Pennovation Works, almost like characters in a video game discovering a new level. “The robots work together to build the map, localize where all the people are and where different landmarks are, which is really important if you're giving directions to first responders.”
How Penn’s experts came together for PRONTO
Reflecting on how the PRONTO team and project came together, Cannon notes the unique advantages that come from working at Penn. “It’s really an embarrassment of riches,” he says. “Sometimes you don’t even realize the level of expertise that exists just across the street.”
Cannon, Eaton, and Qasim first connected because they were on teams applying for the same grants. “It’s a small enough environment that eventually, you cross paths with the right people,” Cannon says. “And once you do, the possibilities are endless.”
Cannon attributes the team’s success in part to Penn’s collaborative environment, where experts from diverse fields can come together to tackle complex problems. “You eventually run into folks just through routine daily interactions,” he says. “And that’s what makes this place special, the ability to draw from such a wide range of knowledge and experience to innovate in ways that might not be possible elsewhere.”