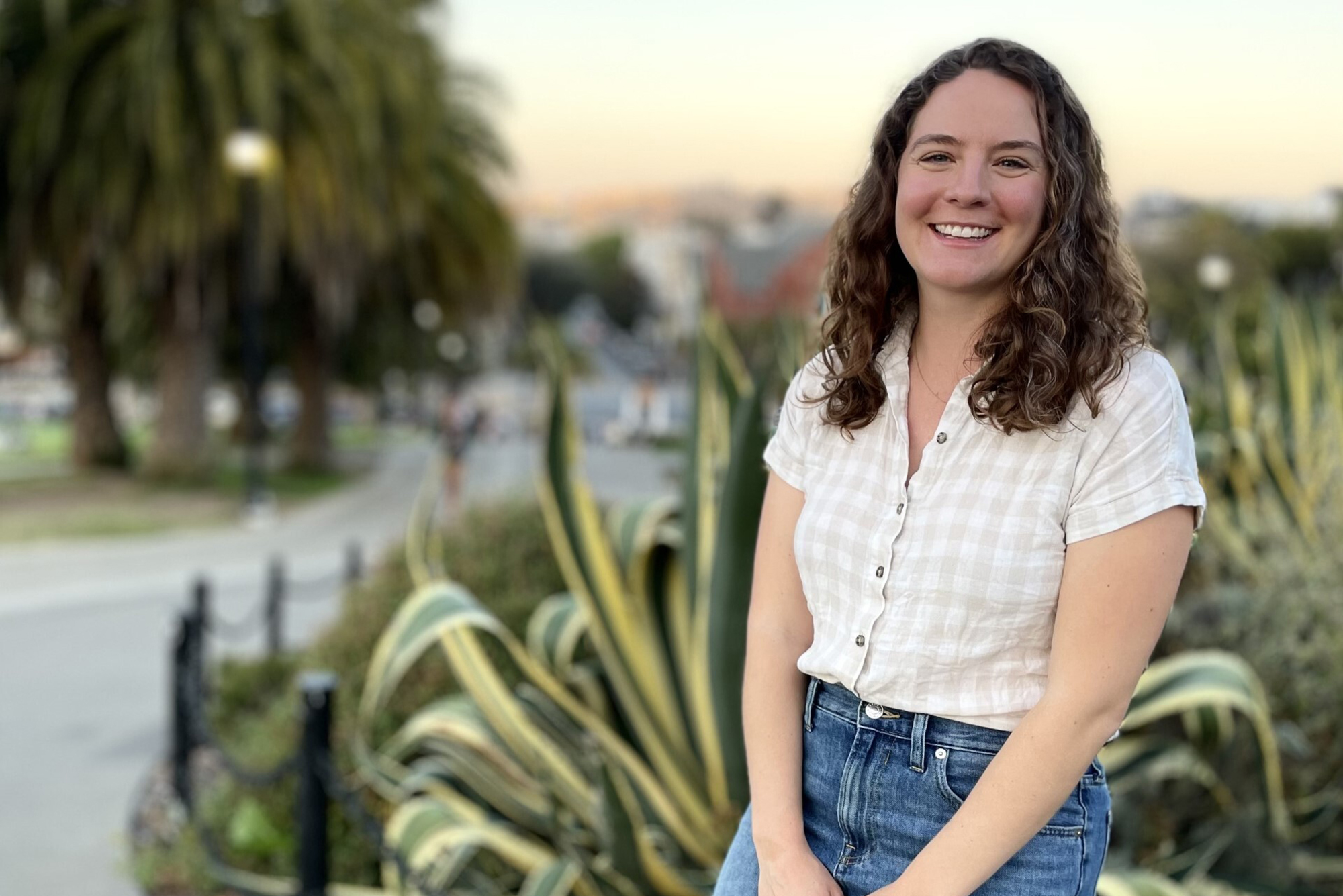
- Who
Clinical psychology Ph.D. candidate Elizabeth (Betsy) Stade has long been interested in questions such as, “What are you thinking? How did you come to think that way? Why do you do the things you do?”
She began studying depression and anxiety as a psychology and religion undergraduate student at Barnard College, work she has expanded upon since coming to Penn’s clinical psychology doctoral program in 2017. Stade says that, beyond being interested in how people perceive the world, she is drawn to the opportunity to help those who are suffering.
Stade has spent the past year doing her clinical residency and will be graduating from Penn this month. Next up is a postdoctoral fellowship at Stanford University with two mentors who both received their doctorates in psychology from Penn: Shannon Wiltsey Stirman and Johannes Eichstaedt.
- What
One question has driven a lot of her work: “How can we measure and detect anxiety and depression using methods other than asking the patient questions or having them fill out a self-report survey?”
In joining Ayelet Meron Ruscio’s Boundaries of Anxiety and Depression Laboratory, Stade says, she was fortunate to have a mentor who supports her interest in novel methodological approaches. As she grew interested in using language to detect anxiety and depression, she asked Lyle Ungar, a computer and information science professor, to be on her dissertation committee.
Working with Ungar and Ruscio, Stade was the lead author on a recently published study in the Journal of Psychopathology and Clinical Science, showing how depression and anxiety have distinct and overlapping language patterns. They found that “I” and “my” usage, words associated with sadness, and lack of positive emotion language were relatively specific to depression, whereas “not” and “no” usage—and a wider array of negative emotion language (words associated with anxiety, stress, and depression)—were relatively specific to anxiety. Using subjects’ interview language, the researchers created machine learning models that could predict—beyond other clinical and demographic factors—anxiety and depression severity.
Stade is also interested in how to best classify anxiety and depression. She says the “categorical and binary approach” of the “Diagnostic and Statistical Manual of Mental Disorders, Fifth Edition” (DSM-5) doesn’t match reality because “we know that anxiety problems cut across disorder categories, and also that severity of anxiety matters, even at low levels. Having mild anxiety, even if it’s below the threshold for a diagnosis, still has negative impacts on a person’s life.”
Stade, Ungar, Ruscio, and Penn psychology professor Robert DeRubeis created a classification system that captures severity of anxiety using four dimensions: intensity, avoidance, pervasiveness, and onset of anxiety. In a paper accepted for publication, they showed the model did no worse than DSM-5 diagnoses in predicting impairment from anxiety, comorbid depression, and suicidal ideation and that the model was simpler.
- Why
“No one likes filling out forms,” Stade says. “It also creates this administrative burden for clinicians as well. I’ve long been asking the question: Can we make this easier on everyone?”
She sees the potential of integrating algorithmic language analysis into clinical practice—with full transparency and consent—and thinks it could help researchers determine how someone’s depression changes during a day or days.
Stade also co-authored a working paper about how large language models like ChatGPT could be applied to psychotherapy. Working in this space, she wants to be both a voice of caution—to communicate why society must tread slowly—“but also to voice excitement about what this could potentially yield in the future.”