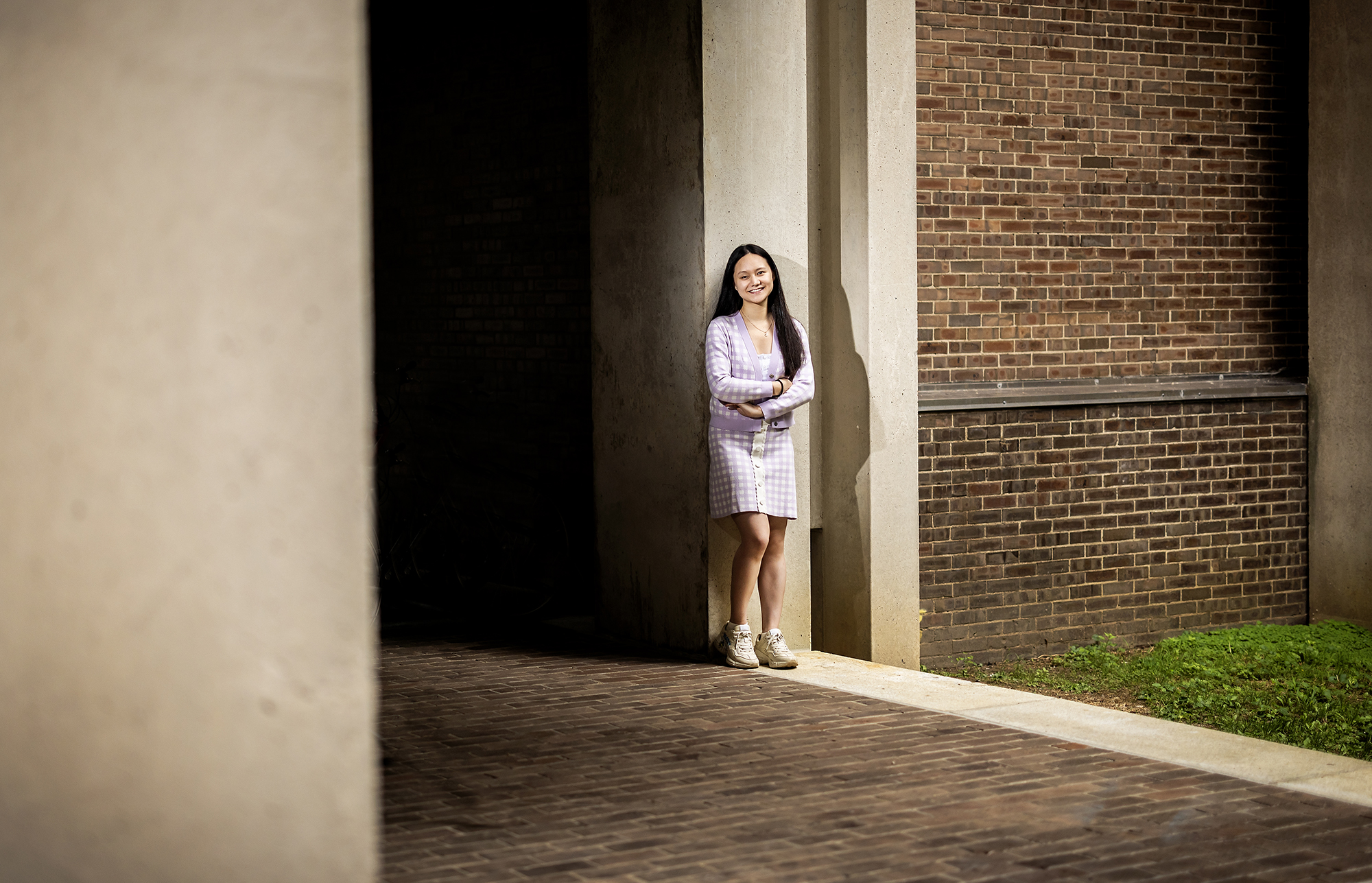
How do people discern a dog’s bark from a crow’s call? Or someone trotting on a cobble-stoned street in wooden clogs versus a pair of sneakers? The answer may seem simple; however, there are a range of frequencies and subtle auditory and temporal cues that allow humans and other animals to identify a suite of features that determine what they may have just heard.
Lily Wei is an undergraduate student who spent the summer conducting research in Vijay Balasubramanian’s Physics of Living Systems Lab and is working on a project that seeks to demystify how the brain deciphers auditory objects like a dog’s bark, a crow’s call, or footsteps in wooden clogs. The effort is supported by the Velay Fellows program and through a College Alumni Society Undergraduate Research Grant, which provides support for undergraduate students conducting research semi-independent or independent research.
Balasubramanian says that a popular example of the problem Wei is trying to solve is related to a phenomenon known as the “cocktail party effect,’ when a listener is bombarded with many overlapping voices but attempts to focus on what one person is saying. “Unlike visual objects, sounds sort of blend together and don’t occlude, rather, they superpose in a way that makes listening to a single voice at a party pretty difficult,” he says.
“The study of how the brain decodes visual stimuli is well developed, so we wanted to take a different approach,” says Wei, from Chongqing, China. “We felt the need to offer a better understanding of how our brains figure out which sound is what, and were quite pleased with our preliminary results.”
To achieve these promising results, Wei, Balasubramanian, and others in the lab like Ronald DiTullio, a recent Ph.D. graduate of the Perelman School of Medicine who led the research, opted for a theoretical approach that sought to reverse engineer the auditory system.
“Dissecting each component of the auditory system was not necessarily feasible and wouldn’t provide us with the information we were looking for concerning the logic of auditory processing,” Wei says. “So, we decided to use algorithms, particularly simple biophysical algorithms rather than the machine-learning methods that are becoming increasingly commonplace.”
She and the other researchers started with a hypothesis for the principles that the auditory system could use to separate categories of sounds, and utilized more direct mathematical methods to understand if this hypothesis could work in the context of natural sounds like animal vocalizations and bird song.
Balasubramanian says that auditory objects, like all objects, are defined by certain kinds of temporal cues, namely coincidence and coherence. Coincidence, in the sense that an object like a book has a specific number of right-angled edges that occur at the same time. That coincidence could have been temporary, but one of the key points is that these coincidences have coherence, or continuity, over time. “Essentially, you could posit that an object is defined by the coincidences that are coherent over time,” he says.
Wei is using temporal learning algorithms that incorporate coincidences and continuities in the signal to learn to identify separate auditory objects in a large variety of sounds from different animals. The algorithms she utilizes go by names like Slow Feature Analysis and Predictable Feature Analysis, which she selected because they offer an elegant way to extract specific features from the auditory objects they analyzed.
“So, one of our goals is to find out if slowness, which is analyzed by the first algorithm, is correlated with predictability, which is analyzed by the second algorithm,” Wei says. “Naturally, you would think if something were slow, it would imply that it’s predictable, but we don’t know if that holds true under our conditions.”
Balasubramanian says that through predictive coding they’ve been studying the predictable elements of natural sounds and have found that the way in which these sounds are decoded based on their predictability matches up very closely with the way in which these sounds are decoded based on their temporal properties.
“We hope to make predictions for how the circuits of the brain would work,” Wei says. “If this is how the circuits in the brain learn to identify objects, by disrupting the auditory signal that's coming to the ear, we should be able to disrupt learning in very specific ways.”
She and Balasubramanian hope that, in making these sorts of theoretical and computational predictions, they may soon collaborate with an experimentalist who can test the ideas experimentally.
“By using simple temporal features, like aspects of continuity and coincidences, you can extract the predictable features that will be useful in guiding an animal’s behavioral outputs, as in, what it will likely do next,” Balasubramanian says.
Vijay Balasubramanian is the Cathy and Marc Lasry Professor in the School of Arts & Sciences’ Department of Physics and Astronomy at the University of Pennsylvania.
Linran (Lily) Wei is a third-year student in the School of Arts and Science at Penn and a Frances Velay Fellow.