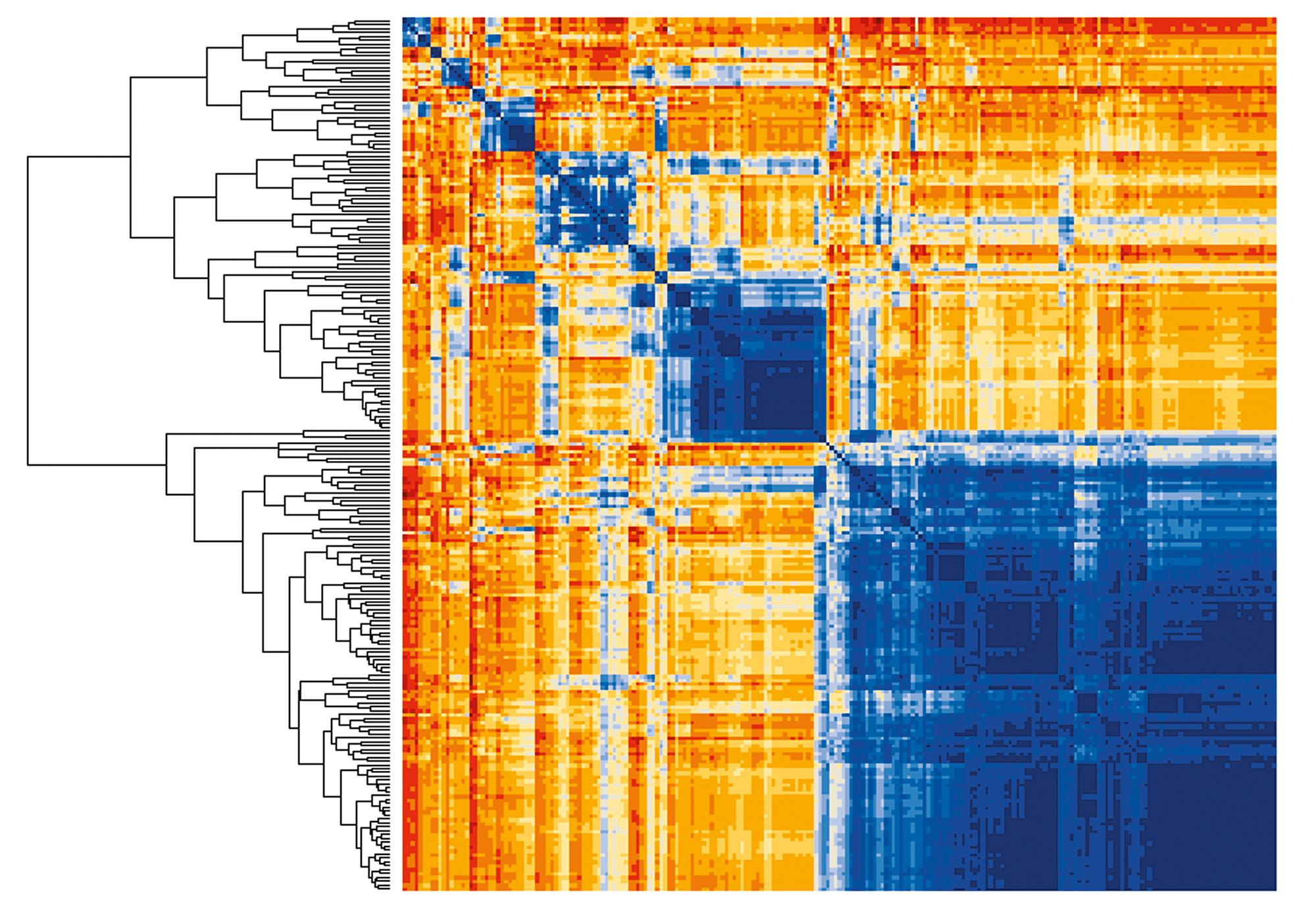
More data is being produced across diverse fields within science, engineering, and medicine than ever before, and our ability to collect, store, and manipulate it grows by the day. With scientists of all stripes reaping the raw materials of the digital age, there is an increasing focus on developing better strategies and techniques for refining this data into knowledge, and that knowledge into action.
Enter data science, where researchers try to sift through and combine this information to understand relevant phenomena, build or augment models, and make predictions.
One powerful technique in data science’s armamentarium is machine learning, a type of artificial intelligence that enables computers to automatically generate insights from data without being explicitly programmed as to which correlations they should attempt to draw.
Advances in computational power, storage, and sharing have enabled machine learning to be more easily and widely applied, but new tools for collecting reams of data from massive, messy, and complex systems—from electron microscopes to smart watches—are what have allowed it to turn entire fields on their heads.
“This is where data science comes in,” says Susan Davidson, Weiss Professor in Computer and Information Science (CIS) at Penn’s School of Engineering and Applied Science. “In contrast to fields where we have well-defined models, like in physics, where we have Newton’s laws and the theory of relativity, the goal of data science is to make predictions where we don’t have good models: a data-first approach using machine learning rather than using simulation.”
Penn Engineering’s formal data science efforts include the establishment of the Warren Center for Network & Data Sciences, which brings together researchers from across Penn with the goal of fostering research and innovation in interconnected social, economic and technological systems. Other research communities, including Penn Research in Machine Learning and the student-run Penn Data Science Group, bridge the gap between schools, as well as between industry and academia. Programmatic opportunities for Penn students include a Data Science minor for undergraduates, and a Master of Science in Engineering in Data Science, which is directed by Davidson and jointly administered by CIS and Electrical and Systems Engineering.
Penn academic programs and researchers on the leading edge of the data science field will soon have a new place to call home: Amy Gutmann Hall. The 116,000-square-foot, six-floor building, located on the northeast corner of 34th and Chestnut Streets near Lauder College House, will centralize resources for researchers and scholars across Penn’s 12 schools and numerous academic centers while making the tools of data analysis more accessible to the entire Penn community.
Faculty from all six departments in Penn Engineering are at the forefront of developing innovative data science solutions, primarily relying on machine learning, to tackle a wide range of challenges. Researchers show how they use data science in their work to answer fundamental questions in topics as diverse as genetics, “information pollution,” medical imaging, nanoscale microscopy, materials design, and the spread of infectious diseases.
Bioengineering: Unraveling the 3D genomic code
Scattered throughout the genomes of healthy people are tens of thousands of repetitive DNA sequences called short tandem repeats (STRs). But the unstable expansion of these repetitions is at the root of dozens of inherited disorders, including Fragile X syndrome, Huntington’s disease, and ALS. Why these STRs are susceptible to this disease-causing expansion, whereas most remain relatively stable, remains a major conundrum.
Complicating this effort is the fact that disease-associated STR tracts exhibit tremendous diversity in sequence, length, and localization in the genome. Moreover, that localization has a three-dimensional element because of how the genome is folded within the nucleus. Mammalian genomes are organized into a hierarchy of structures called topologically associated domains (TADs). Each one spans millions of nucleotides and contains smaller subTADs, which are separated by linker regions called boundaries.
“The genetic code is made up of three billion base pairs. Stretched out end to end, it is 6 feet 5 inches long, and must be subsequently folded into a nucleus that is roughly the size of a head of a pin,” says Jennifer Phillips-Cremins, associate professor and dean’s faculty fellow in Bioengineering. “Genome folding is an exciting problem for engineers to study because it is a problem of big data. We not only need to look for patterns along the axis of three billion base pairs of letters, but also along the axis of how the letters are folded into higher-order structures.”
To address this challenge, Phillips-Cremins and her team recently developed a new mathematical approach called 3DNetMod to accurately detect these chromatin domains in 3D maps of the genome in collaboration with the lab of Dani Bassett, J. Peter Skirkanich Professor in Bioengineering.
“In our group, we use an integrated, interdisciplinary approach relying on cutting-edge computational and molecular technologies to uncover biologically meaningful patterns in large data sets,” Phillips-Cremins says. “Our approach has enabled us to find patterns in data that classic biology training might overlook.”
In a recent study, Phillips-Cremins and her team used 3DNetMod to identify tens of thousands of subTADs in human brain tissue. They found that nearly all disease-associated STRs are located at boundaries demarcating 3D chromatin domains. Additional analyses of cells and brain tissue from patients with Fragile X syndrome revealed severe boundary disruption at a specific disease-associated STR.
“To our knowledge, these findings represent the first report of a possible link between STR instability and the mammalian genome’s 3D folding patterns,” Phillips-Cremins says. “The knowledge gained may shed new light into how genome structure governs function across development and during the onset and progression of disease. Ultimately, this information could be used to create molecular tools to engineer the 3D genome to control repeat instability.”
Chemical and biomolecular engineering: Predicting where cracks will form
Unlike crystals, disordered solids are made up of particles that are not arranged in a regular way. Despite their name, disordered solids have many desirable properties: Their strength, stiffness, smooth surfaces, and corrosion resistance make them suitable for a variety of applications, ranging from semiconductor manufacturing to eyeglass lenses.
But their widespread use is limited because they can be very brittle and prone to catastrophic failure. In many cases, the failure process starts with small rearrangements of the material’s component atoms or particles. But without an ordered template to compare to, the structural fingerprints of these rearrangements are subtle.
“In contrast to crystalline solids, which are often very tough and ductile—they can be bent a lot without breaking, like a metal spoon—we don’t understand how and why nearly all disordered solids are so brittle,” says Rob Riggleman, associate professor in Chemical and Biomolecular Engineering. “In particular, identifying those particles that are more likely to rearrange prior to deforming the material has been a challenge.”
To address this gap in knowledge, Riggleman and his team use machine learning methods developed by collaborators at Penn along with molecular modeling, which allow them to examine in an unbiased fashion a broad array of structural features, identifying those that may contribute to material failure.
“We find machine learning and data science approaches valuable when our intuition fails us. If we can generate enough data, we can let the algorithms filter and inform us on which aspects of the data are important,” Riggleman says. “Our approach is unique because it lets us take a tremendously challenging problem, such as determining in a random-looking, disordered solid, which sections of the material are more likely to fail, and systematically approach the problem in a way that allows physical insight.”
Recently, this approach revealed that softness, quantified on a microscopic structural level, strongly predicts particle rearrangements in disordered solids. Based on this finding, the researchers conducted additional experiments and simulations on a range of disordered materials that were strained to failure. Surprisingly, they found that the initial distribution of soft particles in nanoscale materials did not predict where cracks would form. Instead, small surface defects dictated where the sample would fail. These results suggest that focusing on manufacturing processes that lead to smooth surfaces, as opposed to hard interiors, will yield stronger nanoscale materials.
Moving forward, Riggleman and his team plan to use this information to design new materials that are tougher and less prone to breaking. One potential application is to find greener alternatives to concrete that still have the structural properties that have made it ubiquitous. “The synthesis of concrete releases a large amount of CO2,” Riggleman says. “With the global need for housing growing so quickly, construction materials that release less CO2 could have a big impact on decreasing overall carbon emissions.”
Computer and information science: Navigating information pollution
One unfortunate consequence of the information revolution has been information contamination. These days, it can be difficult to establish what is really known, thanks to the emergence of social networks and news aggregators, combined with ill-informed posts, deliberate efforts to create and spread sensationalized information, and strongly polarized environments. “Information pollution,” or the contamination of the information supply with irrelevant, redundant, unsolicited, incorrect, and otherwise low-value information, is a problem with far-reaching implications.
“In an era where generating content and publishing it is so easy, we are bombarded with information and are exposed to all kinds of claims, some of which do not always rank high on the truth scale,” says Dan Roth, Eduardo D. Glandt Distinguished Professor in Computer and Information Science. “Perhaps the most evident negative effect is the propagation of false information in social networks, leading to destabilization and loss of public trust in the news media. This goes far beyond politics. Information pollution exists in the medical domain, education, science, public policy, and many other areas.”
According to Roth, the practice of fact-checking won’t suffice to eliminate biases. Understanding most nontrivial claims or controversial issues requires insights from various perspectives. At the heart of this task is the challenge of equipping computers with natural language understanding, a branch of artificial intelligence that deals with machine comprehension of language. “Rather than considering a claim as being true or false, one needs to view a claim from a diverse yet comprehensive set of perspectives,” Roth says.
“Our framework develops machine learning and natural language understanding tools that identify a spectrum of perspectives relative to a claim, each with evidence supporting it.”
Along with identifying perspectives and evidence for them, Roth’s group is working on a family of probabilistic models that jointly estimate the trustworthiness of sources and the credibility of claims they assert. They consider two scenarios: one in which information sources directly assert claims, and a more realistic and challenging one in which claims are inferred from documents written by sources.
The goals are to identify sources of perspectives and evidence and characterize their level of expertise and trustworthiness based on past record and consistency with other held perspectives. They also aim to understand where the claim may come from and how it has evolved.
“Our research will bring public awareness to the availability of solutions to information pollution,” Roth says. “At a lower level, our technical approach would help identify the spectrum of perspectives that could exist around topics of public interest, identify relevant expertise, and thus improve public access to diverse and trustworthy information.”
Electrical and systems engineering: Controlling the spread of epidemics
The emergence of COVID-19, along with recent epidemics such as the H1N1 influenza, the Ebola outbreak, and the Zika crisis, underscore that the threat of infectious diseases to human populations is very real.
“Accurate prediction and cost-effective containment of epidemics in human and animal populations are fundamental problems in mathematical epidemiology,” says Victor Preciado, associate professor and graduate chair of Electrical and Systems Engineering. “In order to achieve these goals, it is indispensable to develop effective mathematical models describing the spread of disease in human and animal contact networks.”
Even though epidemic models have existed for centuries, they need to be continuously refined to keep up with the variables of a more densely interconnected world. Toward this goal, engineers like Preciado have recently started tackling the problem using innovative mathematical and computational approaches to model and control complex networks.
Using these approaches, Preciado and his team have computed the cost-optimal distribution of resources such as vaccines and treatments throughout the nodes in a network to achieve the highest level of containment. These models can account for varying budgets, differences in individual susceptibility to infection, and different levels of available resources to achieve more realistic results. The researchers illustrated their approach by designing an optimal protection strategy for a real air transportation network faced with a hypothetical worldwide pandemic.
Moving forward, Preciado and his team hope to develop an integrated framework for modeling, prediction, and control of epidemic outbreaks using finite resources and unreliable data. Although public health agencies collect and report relevant field data, that data can be incomplete and coarse-grained. In addition, these agencies are faced with the challenge of deciding how to allocate costly, scarce resources to efficiently contain the spread of infectious diseases.
“Public health agencies can greatly benefit from information technologies to filter and analyze field data in order to make reliable predictions about the future spread of a disease,” Preciado says. “But in order to implement practical disease-management tools, it is necessary to first develop mathematical models that can replicate salient geo-temporal features of disease transmission.”
Ultimately, Preciado’s goal is to develop open-source infection management software, freely available to the research community, to assist health agencies in the design of practical disease-containment strategies.
“This could greatly improve our ability to efficiently detect and appropriately react to future epidemic outbreaks that require a rapid response,” Preciado says. “In addition, modeling spreading processes in networks could shed light on a wide range of scenarios, including the adoption of an idea or rumor through a social network like Twitter, the consumption of a new product in a marketplace, the risk of receiving a computer virus, the dynamics of brain activity, and cascading failures in the electrical grid.”
Materials science and engineering: Understanding why catalysts degrade
The presence of a metal catalyst is often necessary for certain chemical reactions to take place, but those metals can be rare and expensive. Shrinking these metals down to nanoparticles increases their ratio of surface area to volume, reducing the overall amount of metal required to catalyze the reaction.
However, metal nanoparticles are unstable. A process called “coarsening” causes them to spontaneously grow by bonding with other metal atoms in their environment. Though the exact mechanism by which coarsening occurs is unknown, the loss of nanoparticles’ surface area advantage has clear consequences, such as the irreversible degradation in the performance of several important systems, including automotive catalytic converters and solid oxide fuel cells.
“This process is bad, as it decreases the efficiency of the catalysts overall, adding significant cost and leading to efficiency losses,” says Eric Stach, professor in Materials Science and Engineering and director of the Laboratory for Research on the Structure of Matter (LRSM). “By gathering streams of rich data, we can now track individual events, and from this, learn the basic physics of the process and thereby create strategies to prevent this process from occurring.”
The Stach lab uses in situ and operando microscopy techniques, meaning it collects data from materials in their native environments and as they function. Advances in electron microscopy techniques have increasingly shed light on how materials react under the conditions in which they are designed to perform; in situ electron microscopy experiments can produce hundreds of high-resolution images per second.
“It is possible for us to gather up to four terabytes in just 15 minutes of work. This is the result of new capabilities for detecting electrons more efficiently,” Stach explains. “But this is so much data that we cannot process it by hand. We have been increasingly utilizing data science tools developed by others in more directly related fields to automate our analysis of these images.”
In particular, Stach and his team have applied neural network models to transmission electron microscopy images of metal nanoparticles. The use of neural networks allows for the learning of complex features that are difficult to represent manually and interpret intuitively. Using this approach, the researchers can efficiently measure and track particles frame to frame, gaining insight into fundamental processes governing coarsening in industrial catalysts at the atomic scale.
The next step for the researchers will be to compare the high-resolution image analyses to computational models, thereby shedding light on the underlying physical mechanisms. In the end, understanding the processes by which these metallic particles coarsen into larger structures may lead to the development of new materials for electronic devices, solar energy and batteries.
“The development of new materials drives nearly all of modern technology,” Stach says. “Materials characterization such as what we are doing is critical to understanding how different ways of making new materials lead to properties that we desire.”
Mechanical engineering and applied mechanics: Developing digital twins
Using powerful magnets and software, a 4D flow MRI can provide a detailed and dynamic look at a patient’s vascular anatomy and blood flow. Yet this high-tech device is no match for a $20 sphygmometer when it comes to measuring one of the most critical variables for heart disease and stroke: blood pressure. Although digital models could be used to predict blood pressure from these high-tech scans, they still have not made their way into clinical practice, primarily due to their high computational cost and noisy data.
To address this problem, Paris Perdikaris, assistant professor in Mechanical Engineering and Applied Mechanics, and his collaborators recently developed a machine learning framework that could enable these sorts of predictions to be made in an instant.
By capturing the underlying physics at play in the circulatory system, for example, a relatively small number of biometric data points collected from a patient could be extrapolated out into a wealth of other vital statistics. This more comprehensive simulation of a patient, nicknamed a “digital twin,” would give a multidimensional view of their biology and allow clinicians and researchers to virtually test treatment strategies.
“Integrating machine learning and multiscale modeling through the creation of virtual replicas of ourselves can have a significant impact in the biological, biomedical, and behavioral sciences,” Perdikaris says. “Our efforts on digital twins aspire to advance healthcare by delivering faster, safer, personalized and more efficient diagnostics and treatment procedures to patients.”
Perdikaris’s team recently published a study showing how this framework, known as “Physics-Informed Deep Operator Networks” can be used to find the relationship between the inputs and outputs of complex systems defined by a certain class of mathematical equations.
Other machine learning systems can discover these relationships, but only through brute force. They might require data from tens of thousands of patients to be properly calibrated, and then would still require significant computational time to calculate the desired outputs from a new patient’s input.
Physics-Informed Deep Operator Networks can tackle this problem in a more fundamental way: One designed to predict blood pressure from blood velocity measured at a specific point in the circulatory system, for example, would essentially learn the underlying laws of physics that govern that relationship. Armed with that knowledge and other relevant variables for a given patient, the system can quickly calculate the desired value based on those fundamental principles.
Moving forward, Perdikaris and his team plan to apply their computational tools to develop digital twins for the human heart, and for blood circulation in placental arteries to elucidate the origins of hypertensive disorders in pregnant women. “Creating digital twins can provide new insights into disease mechanisms, help identify new targets and treatment strategies, and inform decision-making for the benefit of human health,” Perdikaris says.
This article is by Janelle Weaver.